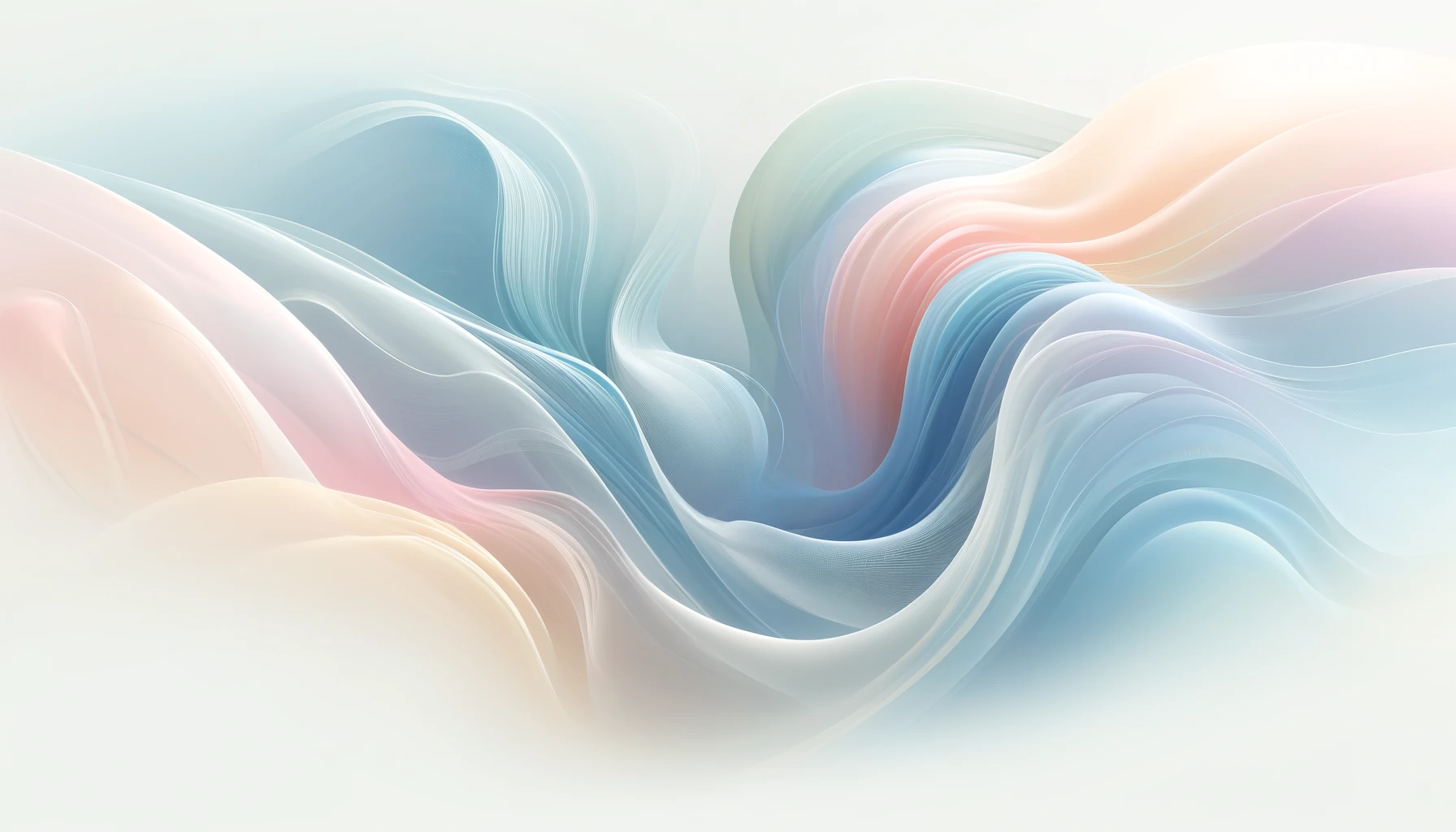
Towards Agentic AI for Science: Hypothesis Generation, Comprehension, Quantification, and Validation (ICLR, 2025)
April 27-28, 2025 | Singapore Expo
About the Workshop
Our mission is to foster interdisciplinary collaboration to develop fully autonomous AI systems, addressing challenges like benchmark datasets, human-AI collaboration, robust tools and methods for validating AI outputs, and trustworthiness. By tackling these issues, we can unlock AI's transformative potential in research. In this workshop, themed Agentic AI for Science, we will explore these critical topics and welcome diverse perspectives. We will focus on integrating agentic AI systems to enhance scientific discovery while upholding rigorous standards. For AI to contribute effectively, it must generate novel hypotheses, comprehend their applications, quantify testing resources, and validate feasibility through well-designed experiments. This workshop serves as a vital forum for collaboration and knowledge-sharing aimed at redefining the landscape of scientific discovery. This workshop aims to address four main research thrusts to propel future research, including (non-exclusively):
Thrust 1. Design and development of agentic AI systems for scientific discovery. The emergence of agentic AI, powered by foundation models—particularly generative models—opens up unprecedented opportunities for scientific discovery. These systems can potentially revolutionize various aspects of the scientific process, including hypothesis generation, comprehension of complex scientific phenomena, quantification, and validation. Designing and developing effective agentic AI systems for scientific discovery is both exciting and non-trivial. Pioneering work in this field has already demonstrated the promise of leveraging scientific tools, agents, and knowledge graphs. Notable examples include ChemCrow, which showcases the potential of AI in chemistry; Crispr-GPT, which applies AI to genetic engineering; and SciAgents , which illustrates the power of multi-agent systems in scientific discovery. These groundbreaking studies highlight the transformative potential of agentic AI in accelerating scientific progress and opening new avenues for research. Key research topics in this thrust include (but not limited to):
- Developing scientific foundation models: Tailoring general foundation models specifically for various scientific fields to enhance relevance and accuracy.
- Effective scientific tool augmentation: Enhancing existing scientific tools and methodologies with agentic AI capabilities.
- Multi-agent decomposition design: Developing frameworks for scientific hypothesis generation using multiple specialized AI agents.
- Human-in-the-loop agentic systems: Improving reliability and interpretability of AI-driven scientific discoveries through strategic human intervention.
Thrust 2. Theoretical foundation for scientific agentic AI. Developing agentic scientific AI requires methods to quantify the predictions and performance of these systems, as well as to validate the scientific hypotheses they generate. A thorough investigation of agentic scientific AI systems also demands solid theoretical foundations and tools to ensure guarantees on their behavior. To analyze and evaluate such systems, we will incorporate theoretical tools in modeling, logical reasoning, model validation and diagnosis, interpretable AI, and other general methods that can provide guarantees on agentic systems. Key topics in this area include, but are not limited to, the following:
- Theoretical foundation: Statistical models and theories of agentic scientific AI, such as theoretical studies on in-context learning, multi-agent communications, game theory, physics-informed hard and soft optimization constraints, and neural operators.
- Logic reasoning: Inductive, deductive, and abductive reasoning; Bayesian reasoning and probabilistic programming; neural-symbolic approaches.
- Model quantification, validation, diagnosis: Theory-driven metrics for quantifying AI system performance; self-evaluation of LLMs; data valuation and data-centric AI; diagnostics for data, architecture, and training processes; creation of standardized benchmarks for evaluating the validity of scientific hypothesis generation; scientific facts and hallucination.
- Interpretable AI: Approaches for explaining agentic AI system behaviors; quantifying trust, safety, and transparency; mechanistic interpretability.
Thrust 3. Practical application of scientific agentic AI. Deploying agentic AI systems in practical scientific research across diverse domains presents numerous challenges, particularly due to the need for domain-specific adaptation such as the unique data formats and model constraints of each scientific field. Bias in training data poses a significant risk, especially in sensitive domains like medicine. Trustworthiness and explainability are essential for scientists to confidently integrate AI-generated hypotheses and solutions into their research. Furthermore, ethical considerations arise when AI systems potentially automate research decisions that may impact public health, policy, or environmental outcomes, underscoring the importance of responsible AI deployment in science.
- Domain-specific model adaptation: Adapting agentic AI models to handle domain-specific data formats, workflows, and tools across various scientific fields; transfer learning and data-efficient fine-tuning.
- Bias detection and mitigation: Identifying and mitigating bias in training data, model design and outputs; fairness-aware AI systems for sensitive domains like healthcare and social science.
- Robustness, trustworthiness and explainability: Methods for improving the transparency and explainability of agentic AI systems in scientific research; uncertainty interpretation and quantification.
- Ethical considerations and responsible use of agentic AI in sensitive research areas; development of AI governance models to ensure accountability and human oversight in automated scientific workflows.
Thrust 4. Open problems and challenges on scientific agentic AI. Despite the promising potential of agentic AI in scientific discovery, many open problems and challenges remain to be addressed. These may include:
- Automatic curation of domain-specific scientific domains and integration of the knowledge into agentic AI systems.
- Advanced mechanisms of multi-agent collaboration in scientific discovery, with considerations of their scalability and computational efficiency.
- Continual evolution and learning of agentic AI systems; Mechanisms for updating models and improving performance based on experimental results, new data and discoveries.
- Validation and reproducibility of results generated by agentic AI systems.
Call for Papers
We are pleased to announce the Workshop on Agentic AI for Science: Hypothesis Generation, Comprehension, Quantification, and Validation at The International Conference on Learning Representations (ICLR) 2025, to be held at Singapore Expo, Singapore, on April 27-28, 2025. This workshop aims to explore the transformative potential of agentic AI in scientific discovery, focusing on hypothesis generation, validation, and other critical stages of the scientific process. By fostering interdisciplinary collaboration, the workshop seeks to address challenges and unlock new opportunities in the design and application of agentic AI systems.
Workshop Themes
We invite contributions addressing the following research thrusts:
- Design and Development of Agentic AI Systems: Exploring frameworks, tools, and human-in-the-loop systems for scientific discovery.
- Theoretical Foundations: Developing statistical models and reasoning approaches for hypothesis validation and performance assessment.
- Practical Applications: Examining domain-specific adaptations, ethical considerations, and governance frameworks for responsible deployment.
- Open Problems and Challenges: Addressing issues in knowledge integration, validation, and continual improvement of agentic AI systems.
Key Focus Areas
Submissions are encouraged in the following areas (not exhaustive):
- AI-driven hypothesis generation and validation.
- Statistical and logical reasoning approaches.
- Applications of AI in scientific experimentation.
- Ethical, reproducibility, and governance challenges in AI-driven science.
Types of Contributions
Our proposed workshop invites a diverse array of paper types, including original research, position papers, short/tiny papers and survey articles, all aimed at advancing Agentic AI research for scientific discovery.
- Original research papers: Present groundbreaking findings, innovative methodologies, or theoretical insights.
- Position papers: Provide thought-provoking perspectives on emerging trends and challenges in the field.
- Short/Tiny Papers Track: This track encourages submissions that are innovative and exploratory, even if not yet fully developed. We invite concise, impactful contributions with a page length of 5 pages (excluding references), focusing on novel ideas, preliminary results, or insightful perspectives that can spark discussions and inspire further research.
- Survey articles: Offer comprehensive overviews of specific topics, illuminating current research landscapes and proposing future directions.
Future Dates and Deadlines
- Call for Papers released: January 10, 2025
- Submission period: January 10 - February 10, 2025
- Submission Deadline: February 10, 2025 (Anywhere on Earth, AoE)
- Review period: February 10 - March 02, 2025
- Notification of Acceptance: March 04, 2025
- Camera-Ready Submission: March 20, 2025
- Workshop Dates: April 27-28, 2025
Submission Site
We will use OpenReview to manage the submissions and reviewing process. All listed authors must have an up-to-date OpenReview profile, properly attributed with current and past institutional affiliation, homepage, Google Scholar, DBLP, ORCID, LinkedIn, Semantic Scholar (wherever applicable). The OpenReview profile will be used to handle conflict of interest and paper matching. Submissions will not be made public on OpenReview during the reviewing period.
Abstracts and papers can be submitted through the OpenReview platform: OpenReview Submission Site .
Camera-Ready Submission Guidelines
Authors of accepted papers are required to submit the Camera-Ready version through the OpenReview Platform . The final submission must incorporate all necessary elements, including implementation code, author affiliations, and address the reviewer comments. Additionally, authors must provide a 3-5 minute video recording summarizing their paper along with presentation slides. These materials should be uploaded to the "[ Event Metadata for Authors (e.g., Poster and Slide Uploads...) ]" section corresponding to their paper on the ICLR Agentic AI for Science Workshop Page.
Poster Guidelines
- Workshop Poster size: Maximum size allowed for printing: 61cm x 91cm (24in x 36in) width/height.
- Poster Printing: You can print your poster using any service you want or ICLR offers an optional poster printing service with delivery to the convention center. Please use the Workshop poster order form to make the order.
Submission Guidelines
Submissions must adhere to the ICLR 2025 submission format and be submitted as PDFs through the OpenReview platform. All submissions will undergo a rigorous double-blind peer review process. Accepted papers will be included in the ICLR 2025 workshop proceedings.
- Deadlines: Submission deadlines are strict, and no extensions will be granted. Placeholder/dummy abstracts are not allowed.
- Tiny Papers Track: This year, ICLR is discontinuing the separate "Tiny Papers" track, and is instead requiring each workshop to accept short (3–5 pages in ICLR format, exact page length to be determined by each workshop) paper submissions, with an eye towards inclusion; see Call For Tiny Papers for more details. Authors of these papers will be earmarked for potential funding from ICLR, but need to submit a separate application for Financial Assistance that evaluates their eligibility. This application for Financial Assistance to attend ICLR 2025 will become available on ICLR Website at the beginning of February and close on March 2nd.
- Authorship: All listed authors must have contributed substantially to the work and agree to its submission. Large Language Models (LLMs) cannot be listed as authors but may be cited as tools if used appropriately.
- Anonymity: Submissions must follow the double-blind review process. Authors should ensure that no identifying information appears in the paper, supplementary material, or any external links.
-
Formatting: Submissions must use the ICLR 2025 LaTeX template. Papers are limited to 9 pages of content, with unlimited space for references and an optional appendix. The main content of the paper (first 9 pages) must be self-contained, as reviewers are not required to read beyond these.
The LaTeX template is available for download: ICLR 2025 LaTeX Template. - Originality: Submissions must present original work. Papers under review at, or published in, other peer-reviewed venues cannot be submitted. Previously presented work in workshops or non-archival formats is allowed but must be appropriately anonymized. For details, visit the ICLR 2025 Author Guide.
- Ethics: Authors are encouraged to include a section on the ethical implications of their research and data use. Submissions must comply with ICLR’s ethics policies, available at ICLR Code of Ethics.
- Code of Conduct: Authors are required to adhere to ICLR’s code of conduct, available at ICLR Code of Conduct.
Reviewing Process
Each submission will undergo a rigorous double-blind peer review process. Submissions will be evaluated on criteria such as technical merit, originality, potential impact, and ethics.
Reviewers, including organizers, will not evaluate submissions from individuals who:
- Have been colleagues within the same organization in the past three years.
- Have co-authored publications within the last three years.
- Are currently affiliated with the same institution as the submitting authors.
Publication and Presentation Policies
Accepted papers will be published as part of ICLR 2025 workshop proceedings. Authors of accepted papers are required to present their work at the workshop, either as oral presentations or posters, as determined by the workshop organizers. Papers must be covered by a distinct conference registration and presenters are encouraged to include a clear explanation of the contributions and implications of their work during their presentations. Pre-recorded video presentations may be requested for accessibility and archiving purposes. Virtual presentation options may be provided for authors unable to attend in person, in line with ICLR's hybrid participation policy.
Other AI for Science Workshops at ICLR 2025
If you feel that your work is not a good thematic fit for the Agentic AI for Science workshop, consider submitting to one of the other AI for science workshops, such as:
Program Committee Co-Chairs
-
Dr. Lifu Huang - UC Davis
lfuhuang@ucdavis.edu -
Dr. Dawei Zhou - Virginia Tech
zhoud@vt.edu -
Dr. Adithya Kulkarni - Virginia Tech
aditkulk@vt.edu
Workshop Schedule
The workshop is scheduled to take place during ICLR 2025 on April 27, 2025 in Singapore.
Singapore Time (GMT+8) | Event |
---|---|
08:30 - 08:40 | Opening Remarks (Dr. Lifu Huang) |
08:40 - 09:25 | Keynote (Dr. Marinka Zitnik) |
09:25 - 10:10 | Keynote (Dr. Chandan K Reddy) |
10:10 - 10:55 | Keynote (Dr. Jingrui He) |
10:55 - 11:05 | Coffee Break |
11:05 - 11:50 | Keynote (Dr. Sanmi Koyejo) |
11:50 - 12:35 | Keynote (Dr. Markus J Buehler) |
12:35 - 13:30 | Lunch Break |
13:30 - 14:15 | Keynote (Dr. Michael Mahoney) |
14:15 - 15:15 | Oral Paper Session #1 (12 min talk + 3 min QA) |
15:15 - 15:25 | Coffee Break |
15:25 - 16:15 | Panel Discussion (Dr. Sanmi Koyejo, Dr. Marinka Zitnik, Dr. Yujun Yan, Dr. Beatrice Soh) |
16:15 - 16:45 | Oral Paper Session #2 (12 min talk + 3 min QA) and Interaction Session of Speakers and Presenters with K-12 Students and Graduate Students from Underrepresented Communities from Singapore |
16:45 - 17:45 | Poster Session and Mentoring of K-12 Students and Graduate Students from Underrepresented Communities from Singapore |
17:45 - 18:00 | Closing Remarks with Awards (Dr. Yaoqing Yang, Dr. Yujun Yan, Dr. Beatrice Soh) |
Accepted Papers
Oral Presentations
- MOOSE-Chem: Large Language Models for Rediscovering Unseen Chemistry Scientific Hypotheses
- Large Language Models powered Neural Solvers for Generalized Vehicle Routing Problems
- Agent S: An Open Agentic Framework that Uses Computers Like a Human
- CMAT: A Multi-Agent Collaboration Tuning Framework for Enhancing Small Language Models
- AstroAgents: A Multi-Agent AI for Hypothesis Generation from Mass Spectrometry Data
- ML-Bench: Evaluating Large Language Models and Agents for Machine Learning Tasks on Repository-Level Code
- Neural Nonmyopic Bayesian Optimization in Dynamic Cost Settings
- LLM-Augmented Chemical Synthesis and Design Decision Programs
- Large Language Models Are Innate Crystal Structure Generators
Poster Presentations
- Orchestrating Tool Ecosystem of Drug Discovery with Intention-Aware LLM Agents
- A Simplified a priori Theory of Meaning; Nature Based AI 'First Principles'
- ENHANCING DIVERSITY AND NOVELTY IN TEXT GENERATION VIA MULTI-VIEW EMBEDDINGS
- Evaluation of a Robust Control System in Real-World Cable-Driven Parallel Robots
- Emerging Multi-AI Agent Framework for Autonomous Agentic AI Solution Optimization
- HEP-JEPA: A Foundation Model for Collider Physics
- AgenticHypothesis: A Survey on Hypothesis Generation Using LLM Systems
- Agentic AI for Scientific Discovery: A Survey of Progress, Challenges, and Future Directions
- Automated Machine Learning Research via Agentic Exploration with Human Oversight
- LLM Agents for Literature to Code Conversion: Case Study of Heat Exchanger Design
- MDCROW: Automating Molecular Dynamics Workflows with Large Language Models
- Evolving RL: Discovering New Activation Functions using LLMs
- ProteinHypothesis: A Physics-Aware Chain of Multi-Agent RAG LLM for Hypothesis Generation in Protein Science
- Dynamic Knowledge Integration in Multi-Agent Systems for Content Inference
- APPA : Agentic Preformulation Pathway Assistant
For more details on the accepted papers, visit the OpenReview Workshop page or the ICLR Workshop Page.
ORGANIZERS
Publicity Chair
Workshop Sponsors
Our workshop is sponsored by AIJ and Amazon.
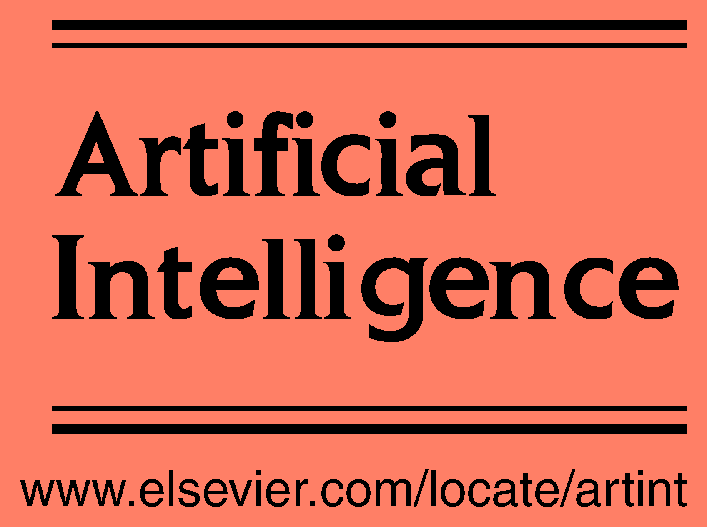
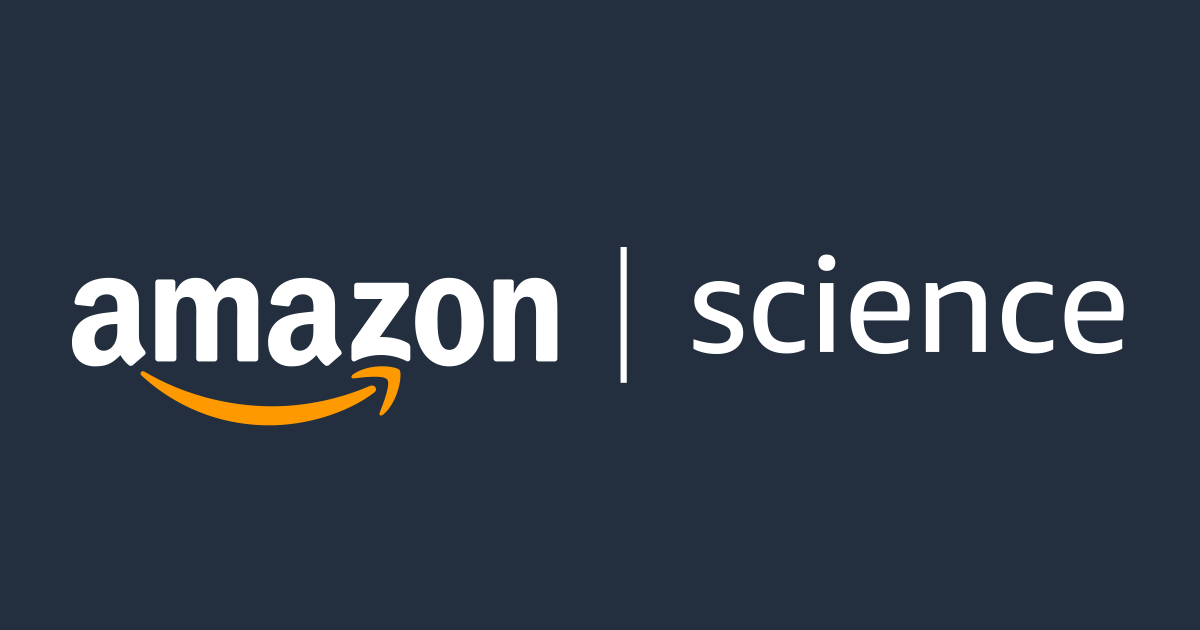
CONTACT US
For inquiries regarding the workshop, please reach out to us at aditkulk@vt.edu.